Some Context: This post is a shortened version of a paper that won the best-paper award at FUN 2020. The paper is ostensibly about trains but the real-world applicability may be somewhat… limited. The actual purpose of the paper is educational: we get to see some of the funnest techniques in probabilistic combinatorics being used to solve a cute problem involving trains.
Part 1: A Consequential Train Ride
A few years ago, while traveling on a train, and on only a few hours of sleep, I was staring out the window. The train crossed a bridge over a road, and the ground was momentarily replaced by a steep drop. Startled, my sleep-deprived mind briefly wondered whether there was still a track underneath us. “Of course there is,” I thought to myself. “Without a track, the train car would have fallen into the gap.”
“Ah, no so fast!” responded the latent mathematician inside me. “If the train car had more than two sets of wheels, then perhaps it could cross the bridgeless gap without falling in.” It was true.
Consider, for example, a train with four sets of wheels: one set in the rear, one set in the front, and one set in each of the first and third quartiles.

As long as the gap in the track is less than the distance between any pair of wheels, then at least three sets of wheels will touch track at all times. Assuming that the center of mass of the train is in the middle half of the train, then the the train does not fall into the gap.
“In fact,” continued the latent mathematician, “what if we have
sets of wheels? Maybe we can build a mono-rail using an asymptotically small amount of track.”
“That’s a stupid idea,” responded I. “Gaps in train track are not something to optimize.”
But I was sleep deprived, so I did it anyway.
The Basic Observation: More Wheels Means Less Track.
Consider a train car with
sets of wheels. Half the sets are evenly dispersed across the first quarter of the train car, and half the sets are evenly dispersed across the final quarter. The train can safely drive down the track as long as at least one set of wheels from each side of the train car is touching track at all times. The train car looks something like this:
Want to build a monorail, but you’re short on track? No problem! You can get away with filling in only an
fraction of the track:

Every quarter of a train length, we place a piece of track whose length is a
fraction of the length of the train car. We get asymptotic cost savings!
To see that this is the best we can do, suppose that the fraction of track that is filled in is less than
, and for symmetry sake suppose the track is circular (i.e., the end of the track loops back to the start). If we place the train at a random position in the circular track, then each wheel has a less than
chance of touching track. By a union bound, it follows that the probability of any wheel in the rear quarter of the train touching the track is less than
. Thus no matter how the track is placed, if the total fraction of track that is filled in is less than
, then there is some position at which the train falls through.
Part 2: Trains with Non-Uniform Wheel Placements
Consider a train car that has
wheels in its rear quarter and
wheels in its front quarter, but suppose that the wheels aren’t evenly spaced. For example, maybe the rear of the car looks something like this:

Can we still fill in an asymptotically small fraction of the track in a way that will allow the train car to drive down the track? In other words, can we place down a small amount of track in a way so that, as the train drives down it there is always at least one wheel from each of the front and rear quarters of the train touching track? It turns out that, via a simple application of the probabilistic method with alterations, we can.
To formalize the situation, let’s focus just on the first quarter of the train. (In particular, up to a constant factor in the amount of train track that we install, we can consider the two quarters of the train separately.) Suppose this portion of the train is
feet long, and assume that each of the
sets of wheels resides at a distinct integer offset from the rear of the train. Our goal is to build a train track that is
feet long. We build the train track out of pillars that are each
foot long and are each placed at integer positions on the track. We are required to put down the pillars in a way so that, as the train drives down the track, at least one wheel from the rear quarter is always touching the track (i.e., touching some pillar). We want to use as little track as possible, with the best we could hope for being a total of
pillars.
As a reminder, there are three variables: the number of wheels
(in the quarter of the train car that we’re considering), the length
of one quarter of the train car, and the length
of the train track. In general, we have
. Note that, although
and
could reasonably be close to one another (e.g.,
), we also want to be able to handle cases where
. This allows for the train car to be configured in truly strange ways — for example, the positions of the wheels could even form a geometric series:

A Randomized Algorithm for Building Track.
Our algorithm for deciding where to put the pillars is a simple example of the probabilistic method with alterations. In particular, we begin by randomly constructing a track that uses only a small number of pillars, and then we show that this track can be slightly altered in order to support (the rear quarter of) the train car at every point. The result will be a train track of length
using only
pillars (and as we shall see later, this is optimal).
We begin by installing each pillar randomly with probability
. Even though this strategy has nothing to do with the structure of where the wheels are on the train, it already does remarkably well. In particular, if we place the train at some given position on the track, then there are
different pillar positions that could potentially hold up the rear quarter. Each of these pillars positions has a
probability of having a pillar installed. It follows that, for a given position on the track, the rear quarter of the train has a

probability of falling through the track. Taking advantage of the identity
, which holds for any
, we see that the wheels fall through the track with probability at most,

In other words, out of all the places we can place the train on the track, only a
-fraction of them will be problematic (in expectation). To fix this, we can just install one additional pillar to remedy each of these problematic positions. The result is a train track that fully supports the rear quarter of our train, and that uses only
total feet of track, in expectation.
The construction is kind of fun to visualize. We start with some random pillars, and then we add additional pillars as needed so that the train never falls through the track:

Of course, our bound of
isn’t quite as good as we did when the wheels were evenly spaced out (we are a roughly
factor worse). But it’s still pretty amazing! No matter how the wheels are distributed across each quarter of the train car, we can get away with installing only a
fraction of the track!
Part 3: A Matching Lower Bound
In the rest of this post, we’re going to prove that the construction above is optimal. (This is where the math gets really cool.) That is, we’ll show that there exists a train car configuration for which
pillars are necessary. We are going to again use the probabilistic method, but this time in a more sophisticated way.
We continue to focus only on the rear quarter of the train car, which is
feet long. We set
, and we will construct the rear quarter of the train car by placing
wheels at integer positions in the set
. We will then consider a track of length
, and show that
pillars are necessary in order to support the rear quarter of the train car at all positions on the track. Recall that each pillar is one foot wide and is placed at an integer offset on the track.
Let
be the set of wheel-positions in the rear quarter of the train car. We choose
by placing a wheel at each position in
independently with probability
. This means that
has
wheels in expectation, but may not actually have exactly
wheels. The important thing to note is that, with at least
probability,
has
or more wheels.
Now consider a track layout given by a subset
of
, and satisfying
. Whereas
is a random variable,
is a fixed set.
Define
to be the event that, for every possible position of the rear quarter of the train on the track, at least one wheel from the rear quarter of the train is supported by track. From the perspective of the train car,
is a good event. Formally,
occurs if for every offset
, we have
.
The key to proving the desired lower bound is to show that the probability of
occurring is very small, namely that
. Because
is a
-element subset of
, there are at most
possibilities for
. Taking a union bound over all of these possibilities implies that
![\displaystyle \Pr[X_{C, T} \text{ for any }T] < \frac{1}{2}.](https://s0.wp.com/latex.php?latex=%5Cdisplaystyle+%5CPr%5BX_%7BC%2C+T%7D+%5Ctext%7B+for+any+%7DT%5D+%3C+%5Cfrac%7B1%7D%7B2%7D.&bg=ffffff&fg=000000&s=0&c=20201002)
On the other hand, we know that the number of wheels
is less than
with probability at most
. By a union bound, the probability that either
has fewer than
wheels or that
holds for some
is less than
. Hence there must exist a car
with
or more sets of wheels, and such that no track
of size smaller than
satisfies
. In fact, with slightly more careful bookkeeping, one can show that an even stronger property is true: almost all choices of how to place
wheels in
require a track of size larger than
to support the car.
To complete the lower bound, the challenge becomes to show that
is very small. That is, for a given choice of track
containing
pillars, the probability that
supports the rear-quarter of the train car
is small.
Rather than examine the event
directly, we instead examine a related quantity. Define
to be the number of positions
for which
(i.e., the number of positions in which the rear quarter of the car, given by
, falls through the track
).
The relationship between
and
is that
occurs if and only if
. Our approach to completing the analysis will be to first show that
is relatively large, and then to show that the probability of
deviating substantially from its expected value is small. This, in turn, implies that
is small, completing the analysis. In other words, the problem of proving that there exists a train-car configuration requiring a large amount of track is reduced to the problem of proving a concentration inequality on the random variable
.
For each position
, the set
consists of
elements. Since each of these elements is contained in
with probability
, the probability that
avoids all of the elements in
is given by,
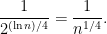
Summing over the values of
, it follows that the expected number of positions
in which
is
![\displaystyle \mathop{\mathbb E}[Y_{C, T}] = 2n \cdot \frac{1}{n^{1/4}} > n^{3/4}.](https://s0.wp.com/latex.php?latex=%5Cdisplaystyle+%5Cmathop%7B%5Cmathbb+E%7D%5BY_%7BC%2C+T%7D%5D+%3D+2n+%5Ccdot+%5Cfrac%7B1%7D%7Bn%5E%7B1%2F4%7D%7D+%3E+n%5E%7B3%2F4%7D.&bg=ffffff&fg=000000&s=0&c=20201002)
The final step of the analysis is to prove a concentration inequality on
. Standard Chernoff bounds do not apply here because
is not a sum of independent indicator random variables. Instead, we employ a more powerful tool, namely McDiarmid’s Inequality:
McDiarmid’s Inequality (1989): Let
be independent random variables over an arbitrary probability space. Let
be a function mapping
to
, and suppose
satisfies,

for all
. That is, if
are fixed, then the value of
can affect the value of
by at most
; this is known as the Lipschitz Condition. Then for all
,
![\displaystyle \Pr[|F(A_1, \ldots, A_m) - \mathop{\mathbb E}[F(A_1, \ldots, A_m)]| \ge R \cdot S] \le 2e^{-2S^2 / m}.](https://s0.wp.com/latex.php?latex=%5Cdisplaystyle+%5CPr%5B%7CF%28A_1%2C+%5Cldots%2C+A_m%29+-+%5Cmathop%7B%5Cmathbb+E%7D%5BF%28A_1%2C+%5Cldots%2C+A_m%29%5D%7C+%5Cge+R+%5Ccdot+S%5D+%5Cle+2e%5E%7B-2S%5E2+%2F+m%7D.&bg=ffffff&fg=000000&s=0&c=20201002)
To apply McDiarmid’s Inequality to our situation, recall that
is defined to be the number of positions in the track
that the rear quarter of the car, given by
, falls through. Whereas the track
is fixed, each of the
possible wheels in
is picked with probability
. Define the indicator random variables
so that
indicates whether
. As required by McDiarmid’s Inequality, the
‘s are independent of one-another, and
is a function of the
‘s.
Now we show that the Lipschitz Condition holds with
. Recall that the track
consists of only
pillars. Out of the
possible wheels
that
could contain, each of those wheels
is only relevant (to the car’s stability) when the car is
feet down the track for some
that places wheel
on top of a pillar. Since there are only
pillars, each wheel
is only relevant to the train car’s stability for
positions
on the track. In other words, for a given wheel position
, there are only
values of
for which
can possibly contain
. As a result, each
can only affect the value of
by at most
, meaning that the Lipschitz Condition holds with
.
Applying McDiarmid’s Inequality, we can deduce that
![\displaystyle \Pr[n^{3/4} - Y_{C, T} > n^{5/8} \cdot (\ln n) / 4] \le 2e^{-n^{1/4}}.](https://s0.wp.com/latex.php?latex=%5Cdisplaystyle+%5CPr%5Bn%5E%7B3%2F4%7D+-+Y_%7BC%2C+T%7D+%3E+n%5E%7B5%2F8%7D+%5Ccdot+%28%5Cln+n%29+%2F+4%5D+%5Cle+2e%5E%7B-n%5E%7B1%2F4%7D%7D.&bg=ffffff&fg=000000&s=0&c=20201002)
When
is large, this probability is much smaller than
. It follows that
.
Summing over all possible options for the track
, the probability that any of them support the train car
is therefore less than
. This completes the proof: we have shown (non-constructively) that some train car
with
or more wheels fails to be supported by any track
consisting of
or fewer pillars.
Conclusion. If you’ve made it to here, you might want to take a look at the paper that this blog post is based on. It turns out that there’s even more fun to be had: in fact, the train-track problem can be used to give illustrative examples of some of the most useful techniques in probabilistic combinatorics, including, e.g., the Lovász Local Lemma and the Method of Conditional Expectations!
1 This research was partially sponsored by the United States Air Force Research Laboratory and was accomplished under Cooperative Agreement Number FA8750-19-2-1000. The views and conclusions contained in this document are those of the authors and should not be interpreted as representing the official policies, either expressed or implied, of the United States Air Force or the U.S. Government. The U.S. Government is authorized to reproduce and distribute reprints for Government purposes notwithstanding any copyright notation herein.